Artificial Intelligence (AI) has reached a pivotal point.
It remained out of the spotlight for years, providing utility to various technologies and innovations, but its control was in the hands of engineers, computer scientists and the like.
Now, AI is available to everyone – those with both good and bad intentions.
In today’s blog, we’ll delve into the dual role of AI in cybersecurity. While AI offers the potential to strengthen automated processes and elevate threat detection capabilities, it also heightens the threat landscape and may pose cybersecurity challenges for organizations.
What is Artificial Intelligence (AI)?
As we progress through the intricate terrain of digital transformation, fresh ideas and technologies consistently come into focus. Among these, generative AI has surfaced as a transformative force in the tech sphere.
However, what distinguishes it from conventional machine learning?
Generative AI
Generative AI, as a concept, has been around for several decades, but its more advanced forms have gained significant attention in recent years.
The term “generative AI” refers to AI systems capable of creating new content that is original and creative, often emulating human-like outputs.
A few examples of generative AI applications include:
- Text Generation: Models like OpenAI’s GPT-3 can produce coherent and contextually relevant written content, aiding in content creation, drafting emails and even coding assistance.
- Art and Design: Generative adversarial networks (GANs) can create unique artwork, providing artists with inspiration or even collaborating with them.
- Image Creation: StyleGAN2 can craft high-resolution images, demonstrating proficiency in generating lifelike faces and other visuals.
While the concept of generative AI has been present for decades, the recent advancements in machine learning techniques and computational resources have propelled it to the forefront of AI research and application.
Traditional Machine Learning
During the 1940s and 1950s, Alan Turing’s contributions to machine learning were foundational in terms of establishing theoretical concepts that underpin modern computational processes and artificial intelligence.
Although the term “machine learning” was not in use during Turing’s time, his work laid the groundwork for the development of algorithms and concepts that are now essential in machine learning research and applications.
Essentially – machine learning is a branch of artificial intelligence that equips computers with the ability to learn from data and improve their performance over time. This technology enables systems to recognize patterns, make decisions and adapt to new information.
Examples of machine learning include:
- Natural Language Processing (NLP): Models, such as BERT, can understand and generate human language, facilitating sentiment analysis, language translation and chatbot interactions.
- Fraud Prevention: Financial transactions can be monitored to identify fraudulent activities, detecting account takeovers and fraudulent credit card transactions.
- Threat Intelligence: Large volumes of data can be analyzed to identify emerging threats and trends, automatically categorizing and prioritizing threat indicators from various sources.
Even though Generative AI and traditional machine learning are related concepts, they serve different purposes within the realm of artificial intelligence.
The Benefits of AI in Cybersecurity
AI’s integration into cybersecurity offers a multitude of benefits that significantly enhance defense strategies.
These advantages not only bolster an organization's security posture, but alleviate several challenges faced by human-operated systems.
Enhanced User Authentication and Access Control
AI can analyze user behavior to establish baseline patterns, making it easier to identify unauthorized access attempts or abnormal activities.
For example, imagine Sally works in the accounting department of a company. As part of her regular job, she frequently accesses specific financial data pages and reports.
One day, the system detects Sally accessing HR and R&D data – unusual for her. It triggers an alert, allowing the security team to swiftly respond and an investigation reveals an account breach.
Machine learning plays a crucial role in recognizing patterns of normal behavior and identifying anomalies that might indicate security threats.
By continuously monitoring employee activities and comparing them to established patterns, machine learning algorithms can help organizations quickly detect and respond to unusual behavior, thereby enhancing data security and mitigating potential risks.
Reduced Reliance on Human Efforts and Human Error
AI automates various security processes, alleviating the burden on security teams and reducing the risk of human errors.
Mundane and repetitive tasks, such as monitoring logs and analyzing vast amounts of data, can be handled by AI algorithms.
This enables human experts to focus on more complex tasks that require critical thinking and strategic decision-making.
Scalable Data Analysis & Advanced Threat Detection
As previously mentioned, the sheer volume of data generated in modern digital environments is often overwhelming.
AI-driven algorithms excel at sifting through enormous datasets, extracting valuable insights and identifying anomalous patterns that might indicate cyber threats. This capability enables swift and accurate threat detection, reducing the time it takes to respond to potential breaches.
Machine learning algorithms can learn from historical data and identify evolving threat vectors. By recognizing subtle correlations and hidden patterns, AI can predict potential vulnerabilities or attacks that might be overlooked by traditional methods.
This proactive approach helps organizations stay ahead of cyber adversaries.
Potential Drawbacks of AI in Cybersecurity
While AI has introduced transformative advancements, its integration also presents a set of potential challenges that organizations must carefully navigate.
Data Privacy Concerns
The complexity of AI algorithms can make them difficult to understand and interpret. Lack of transparency in how AI systems arrive at their decisions can lead to distrust and hinder individuals’ ability to assess privacy risks.
Imagine John, an employee at a large technology company, comes across a generative AI platform that promises to generate creative and innovative content. Intrigued, he decides to use the platform to assist in his work, however, he unknowingly includes proprietary and sensitive company information as input for the generative AI tool.
In doing so, John exposes himself and his organization to a multitude of privacy concerns, including:
- Data Leakage: By inputting proprietary information into a generative AI platform, he inadvertently exposes sensitive data to an external service. This can lead to unauthorized access to company data and the potential leakage of intellectual property.
- Third-Party Access: Generative AI platforms might store input data temporarily for processing. This raises concerns about whether the platform provider or any malicious actors could gain access to the proprietary information, leading to data breaches or corporate espionage.
- Legal and Compliance Issues: Sharing proprietary company data with third-party platforms might violate confidentiality agreements, intellectual property rights and data protection regulations. This could result in legal ramifications and damage to the company’s reputation.
AI-Powered Attacks
Hackers are increasingly leveraging AI techniques to develop sophisticated attacks.
Adversarial machine learning can be used to craft malicious data that can evade detection by AI-based security systems, potentially leading to the creation of AI-generated phishing emails or malware.
To give you a better picture of how these attacks occur, here’s an imaginary scenario:
- Automated Phishing Scheme: The attackers utilize AI to automate the entire phishing process. AI algorithms scan public sources, social media profiles and leaked data to gather information about XYZ Bank’s customers and employees.
- Tailored Attack Content: The AI-generated content is customized for each target, including personal details and context to make the phishing emails seem genuine. The language used in the emails Is carefully crafted by AI to mimic the bank’s communication style.
- Realistic Spoofing: AI helps the attackers perfectly replicate XYZ Bank’s email domain, creating convincing sender addresses that are challenging to distinguish from legitimate bank emails.
- Targeted Timing and Context: The AI determines the optimal time to send phishing emails, taking into account recipients’ time zones and the bank’s communication patterns.
- Response Mimicry: If a recipient responds skeptically, the AI-powered attacker’s system generates responses to address concerns, further enhancing the illusion of legitimacy.
This scenario highlights how AI-powered attacks, like automated phishing campaigns with AI-generated content, can be highly effective in deceiving recipients and causing severe harm to organizations.
Algorithmic Bias and Ethics
As AI continues to shape various facets of modern life, it brings with it a range of potential drawbacks that demand careful attention. These challenges encompass algorithmic bias, concerns about the quality of information AI relies on and the intricacies of judgement calls made by AI systems.
Algorithmic bias refers to the tendency of AI algorithms to produce results that disproportionately favor or disadvantage certain groups or individuals due to biased training data or flawed design.
Additionally, AI’s effectiveness relies on the quality and diversity of its training data. Challenges arise when data is incomplete, outdated or skewed, leading to suboptimal AI performance.
Together, these issues underscore the complexity of integrating AI into the decision-making processes and highlight the need for thoughtful management.
Overreliance on Automation
While AI-driven automation holds the promise of enhancing efficiency and productivity, it also carries the risk of weakening human skills and decision-making capabilities.
When was the last time you sent a document without putting it through spell-check? Can you recall your family’s phone numbers off the top of your head? When was the last time you solved an equation without using a calculator?
Nowadays, almost everything’s automated.
AI’s potential for enfeeblement and overreliance should serve as a reminder that technology augmentation should complement human abilities, rather than replace them.
What Should Your Organization Be Doing?
If you’ve made it this far in the blog, congratulations! You’re probably wondering what your organization can do to proactively safeguard yourself from the potential risks and challenges associated with AI adoption.
Well, here are a few things we recommend:
- Establish a Comprehensive Acceptable Use Policy (AUP). This policy should delineate the ethical boundaries and guidelines for AI deployment, outlining clear directives on data usage, privacy protection, bias mitigation and responsible decision-making.
- Form an AI Steering Committee. To ensure effective oversight, an AI Steering Committee should be formed, comprising experts of various domains to assess AI initiatives, align them with organizational goals and monitor their adherence to ethical standards.
- Implement Robust Risk Management Strategies. These strategies should involve continuous risk assessments, scenario planning and mitigation measures to tackle unforeseen challenges.
Through a combination of these measures, organizations can navigate the AI landscape safely and responsibly. Reach out to a Loffler representative today to get started!
Read Next: Ransomware: Understanding, Educating & Protecting Your Organization
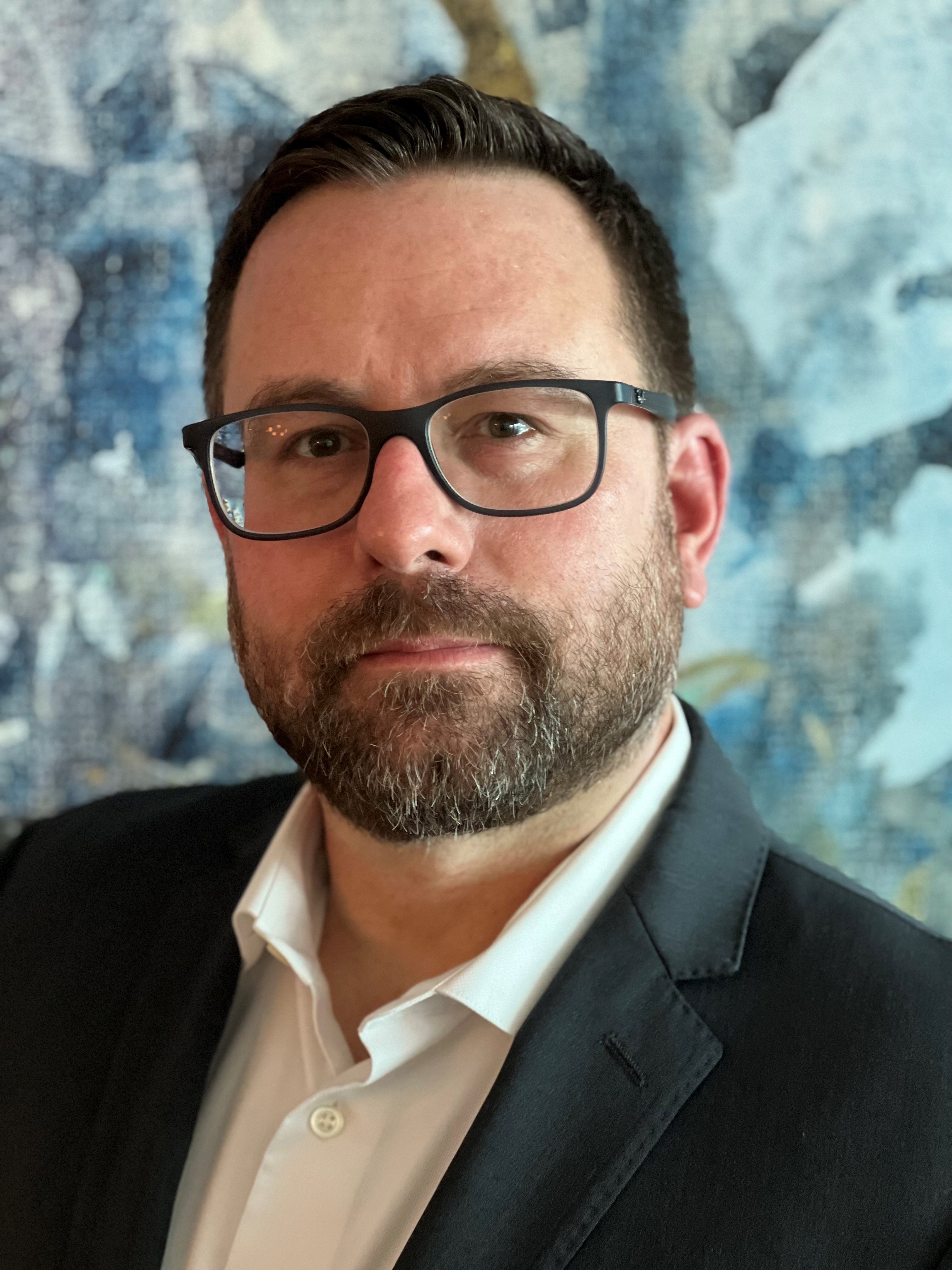
Randy is a CISSP who leads the Cybersecurity and IT Consulting team at Loffler Companies. He is focused on applying his 25+ years of IT experience to help his clients measure, understand and manage information security risk through the vCISO managed consulting program.